The GPT-3 Model: What Does It Mean for Chatbots and Customer Service?
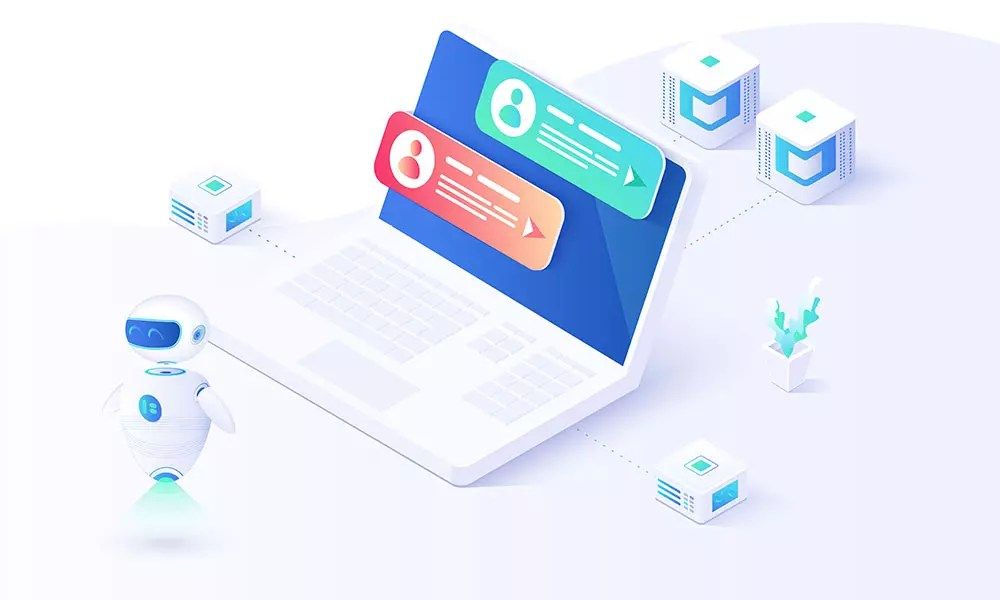
What is GPT-3?
In February 2019, the artificial intelligence research lab OpenAI sent shockwaves through the world of computing by releasing the GPT-2 language model. Short for “Generative Pretrained Transformer 2,” GPT-2 is able to generate several paragraphs of natural language text—often impressively realistic and internally coherent—based on a short prompt.
Scarcely a year later, OpenAI has already outdone itself with GPT-3, a new generative language model that is bigger than GPT-2 by orders of magnitude. The largest version of the GPT-3 model has 175 billion parameters, more than 100 times the 1.5 billion parameters of GPT-2. (For reference, the number of neurons in the human brain is usually estimated as 85 billion to 120 billion, and the number of synapses is roughly 150 trillion.)
Just like its predecessor GPT-2, GPT-3 was trained on a simple task: given the previous words in a text, predict the next word. This required the model to consume very large datasets of Internet text, such as Common Crawl and Wikipedia, totalling 499 billion tokens (i.e. words and numbers).
But how does GPT-3 work under the hood? Is it really a major step up from GPT-2? And what are the possible implications and applications of the GPT-3 model?
How Does GPT-3 Work?
Building GPT-3 required a monumental effort from OpenAI researchers. The details of the GPT-3 model are discussed in the May 2020 paper “Language Models are Few-Shot Learners,” which is 74 pages long and has more than 30 authors. Chuan Li, chief science officer at Lambda Labs, estimates that it would cost $4.6 million, and take 355 years, to run a single training cycle for the GPT-3 model on an NVIDIA Tesla V100 GPU.
Perhaps the most impressive feat of GPT-3 is its ability to generate highly realistic sentences and paragraphs of text. GPT-3’s text generation feature is beyond even the capabilities of GPT-2, which were already a major advancement in the field.
Based on a prompt containing a brief title and subtitle, the GPT-3 model is able to “write” a roughly 200-word news article that appears at least superficially plausible. Below is the GPT-3-generated article that human judges had the most difficulty distinguishing from reality:
Title: United Methodists Agree to Historic Split
Subtitle: Those who oppose gay marriage will form their own denomination
Article: After two days of intense debate, the United Methodist Church has agreed to a historic split – one that is expected to end in the creation of a new denomination, one that will be “theologically and socially conservative,” according to The Washington Post. The majority of delegates attending the church’s annual General Conference in May voted to strengthen a ban on the ordination of LGBTQ clergy and to write new rules that will “discipline” clergy who officiate at same-sex weddings. But those who opposed these measures have a new plan: They say they will form a separate denomination by 2020, calling their church the Christian Methodist denomination.
Note that this article is based on a kernel of truth: in January 2020, the United Methodist Church proposed a split as a result of disagreements over LGBT issues such as same-sex marriage. This seeming verisimilitude was likely key to how this passage convinced so many judges. However, GPT-3’s generated article gets a few notable facts wrong: the name of the new denomination has not been suggested, the proposal was not made at the church’s General Conference, and the Washington Post citation is not based on a real quote.
Perhaps even more impressive, though, is GPT-3’s performance on a number of common tasks in natural language processing. Even compared with GPT-2, GPT-3 represents a significant step forward for the NLP field. Remarkably, the GPT-3 model can demonstrate very high performance, even without any special training or fine-tuning for these tasks.
For one, GPT-3 achieves very strong performance on “cloze” tests, in which the model is tasked with filling in the blank words in a sentence. Given the sentence below, for example, most people would insert a word such as “bat” in the blank space:
George bought some baseball equipment: a ball, a glove, and a _____.
The GPT-3 model can also easily adapt to new words introduced to its vocabulary. The example below demonstrates how, given a prompt that defines the new word, GPT-3 can generate a plausible sentence that even uses the word in past tense:
Prompt: To “screeg” something is to swing a sword at it. An example of a sentence that uses the word screeg is:
Answer: We screeghed at each other for several minutes and then we went outside and ate ice cream.
Surprisingly, GPT-3 is also able to perform simple arithmetic with a high degree of accuracy, even without being trained for this task. With a simple question such as “What is 48 plus 76?” GPT-3 can supply the correct answer almost 100 per cent of the time with two-digit numbers, and roughly 80 per cent of the time with three-digit numbers.
What Does GPT-3 Mean, in General?
In the weeks since the release of GPT-3, many experts have discussed the impact that the model might have on the state of deep learning, artificial intelligence, and NLP.
First, GPT-3 demonstrates that it’s not necessary to have a task-specific dataset, or to fine-tune the model’s architecture, in order to achieve very good performance on specific tasks. For example, you don’t need to train the model on millions of addition and subtraction problems in order to get the right answer to a math question. Essentially, GPT-3 achieved its strong results primarily through brute force, scaling up the model to an incredible size.
This approach has earned mixed reviews from analysts. According to UCLA assistant computer science professor Guy Van den Broeck, the GPT-3 model is analogous to “some oil-rich country being able to build a very tall skyscraper.” While acknowledging the knowledge, skill, and effort required to build GPT-3, Van den Broeck claims that “there is no scientific advancement per se,” and that the model will not “fundamentally change progress in AI.”
One issue is that the raw computing power required to train models like GPT-3 is simply out of reach for smaller companies and academia. Deep learning researcher Denny Britz compares GPT-3 to a particle collider in physics: a cutting-edge tool only accessible to a small group of people. However, Britz also suggests that the computing limitations of less well-endowed researchers will be a net positive for AI research, forcing them to think about why the model works and alternative techniques for achieving the same effects.
Despite the impressive results, it’s not entirely clear what’s going on with GPT-3 under the hood. Has the model actually “learned” anything, or is it simply doing very high-level pattern matching for certain problems? The authors note that GPT-3 still exhibits notable weaknesses with tasks such as text synthesis and reading comprehension.
What’s more, is there a natural limit to the performance of models like GPT-3, no matter how large we scale them? The authors also briefly discuss this concern, mentioning the possibility that the model “may eventually run into (or could already be running into) the limits of the pretraining objective.” In other words, brute force can only get you so far.
Unless you have a few hundred spare GPUs lying around, the answer to these questions will have to wait until the presumed release of GPT-4 sometime next year.
What Does GPT-3 Mean for Customer Service?
Although there’s still much more to learn about how GPT-3 works, the release of the model has wide-ranging implications for a number of industries—in particular, chatbots and customer service. The ability of GPT-3 to generate paragraphs of seemingly realistic text should appeal to anyone interested in creating more convincing, “human-like” AIs.
Tech companies have tried for years to build chatbots that can effectively simulate conversations with their human interlocutors. Yet despite their best efforts, chatbots still aren’t able to simulate the conversational fluency and knowledge of a real human being over a sustained period of time. According to a 2019 survey, 86 per cent of people prefer to speak with humans instead of chatbots, and 71 per cent say they would be less likely to use a brand if there were no human agents available.
Of course, GPT-3 was trained to generate articles and text, not to have a lifelike conversation. But there are indications that models like GPT-3 are approaching human-like language abilities—at least for shallow interactions, as would be involved in a chatbot conversation. The GPT-3 authors found that human judges could only identify the model’s fake articles 52 per cent of the time, which is little better than chance.
It’s not only the realism of GPT-3, but also the advanced tasks it’s able to perform, that differentiate it from the current field of chatbots. Many chatbots on companies’ websites are simply intended as a customer service quality filter, suggesting some common solutions for users before transferring them to a human agent if necessary.
Meanwhile, in terms of natural language processing, GPT-3 is much closer to an “artificial general intelligence” than any chatbot built thus far (although it’s still far from a true AGI). It’s conceivable that one day, highly advanced models like GPT-3 could parse users’ complex queries and solve their problems automatically, without a human agent ever needing to step in.
Furthermore, groundbreaking conversational AIs such as Google’s Meena and Facebook’s BlenderBot, both released in 2020, have also demonstrated that the “brute force” approach is effective when applied specifically to chatbots. Meena and BlenderBot have 2.6 billion and 9.4 billion parameters, respectively, which are only tiny fractions of GPT-3’s 175 billion. It may only be a matter of time before these models pass the Turing test by expanding to the scale of GPT-3, making them virtually indistinguishable from humans in short text conversations.
OpenAI hasn’t yet released the full model or source code for GPT-3, as they did gradually with GPT-2 last year. This puts GPT-3 out of reach for any companies interested in the model’s practical applications (at least for now). But this isn’t the last we’ll hear about GPT-3 by a long shot. We live in exciting times—and whatever research comes next down the pipeline, it will be sure to advance our understanding of the capabilities (and limits) of AI.
Image: Graf Vishenka/Shutterstock