Building a Greener Future: The Importance of Sustainable AI
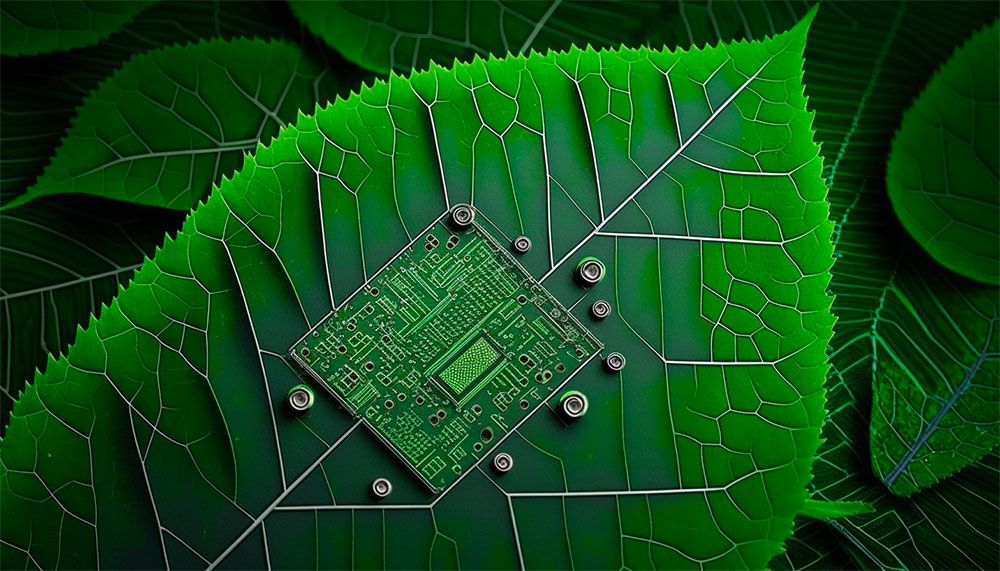
As artificial intelligence (AI) continues to advance and transform industries, there is a growing need to ensure its development and deployment are sustainable and environmentally responsible. While sustainable AI solutions hold great promise for reducing energy consumption, optimising resource use, and addressing pressing environmental challenges, they can also have unintended consequences that must be carefully considered.
The importance of sustainable AI cannot be overstated, particularly in the face of the urgent environmental challenges we currently face. As AI continues to grow in popularity and use, we must address the environmental impact of this technology and ensure that it is deployed sustainably and responsibly.
As a futurist, I believe the sustainability issues of AI and how AI can be used to have a positive impact on the environment are crucial. The purpose is to shed light on the potential challenges and opportunities of sustainable AI and offer insights into how we can create AI systems that promote long-term sustainability and well-being for all.
The Importance of Sustainable Artificial Intelligence
As we embark on a new year, the issue of climate change continues to loom large on the global agenda. With pressure mounting on companies to reduce their carbon footprint and make their environmental impact as small as possible, there is a growing recognition that we need to find innovative solutions to address this urgent challenge. One technology that has the potential to both benefit and harm sustainability efforts is artificial intelligence.
On the one hand, AI and its supporting infrastructure, such as cloud networks and edge devices, consume significant amounts of power and resources. AI has the potential to significantly increase energy consumption and greenhouse gas emissions, particularly if it is not deployed in an energy-efficient manner. This could exacerbate the already dire climate crisis, making it all the more urgent that we address the environmental impact of AI. This raises concerns about AI's environmental impact, particularly given this technology's rapid growth in recent years.
However, there is growing recognition that AI can play a crucial role in enhancing sustainability efforts. By identifying sources of waste and inefficiency in product and infrastructure development, sustainable AI can help companies reduce their environmental impact. Efforts to use more green energy in AI infrastructure are already underway, with companies exploring using renewable energy sources to power their data centres and other AI-related infrastructure.
Sustainable AI can also help address environmental challenges in a more proactive and effective way. For example, we can quickly and effectively identify and respond to environmental threats by using AI to monitor deforestation and illegal fishing.
As we look ahead to 2023, it is clear that there will be a continued focus on using AI to address environmental challenges rather than just boosting corporate profits. Sustainable AI is essential if we are to create a more sustainable future for all. This will require a deep dive into sustainable AI, exploring this technology's trade-offs and potential benefits. By embracing sustainable AI practices, we can harness the power of this technology to create a more sustainable future for all while minimising its negative impact on the planet.
The Environmental Impact of AI
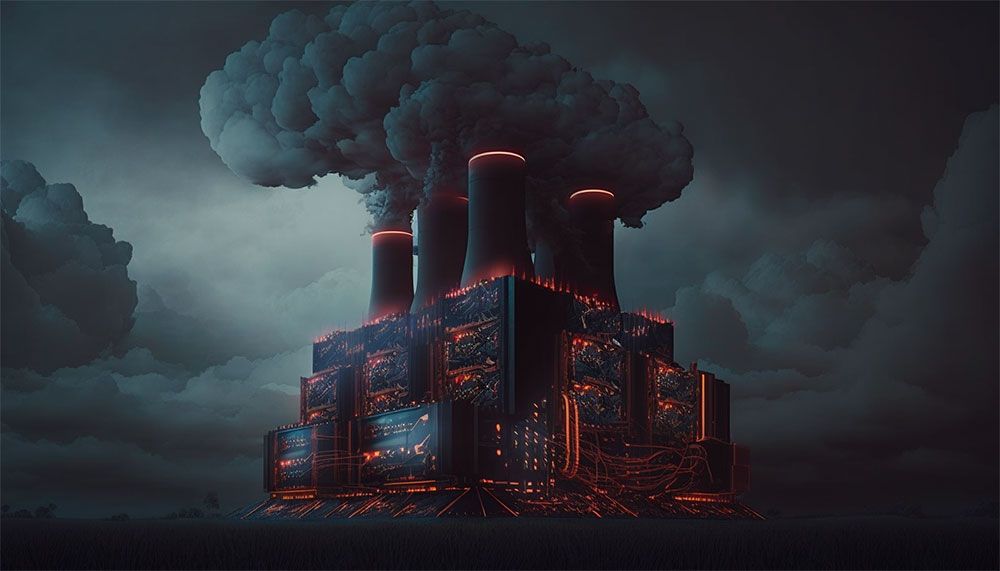
AI has enormous potential to address sustainability challenges such as climate change, sustainable transportation, agriculture, and circular economy practices. However, developing and deploying AI technologies also have significant environmental implications, including high energy consumption, greenhouse gas emissions, and electronic waste.
In this section, we will explore the environmental impact of AI and discuss strategies to mitigate its impact and promote sustainability in AI development and deployment.
AI's Carbon Footprint
AI relies heavily on its supporting infrastructure, including data centres, cloud networks, and edge devices, which consume significant energy and resources. The energy consumption of AI is a significant concern, particularly given the exponential growth of data and the need for ever more powerful computing resources with ever larger neural networks or large language models (LLM) being created. The energy requirements of AI are significant not only in terms of carbon emissions but also in terms of the resources required to build and maintain the necessary infrastructure.
The computing power required for AI applications and the infrastructure that supports them consume large amounts of electricity and other resources, resulting in substantial carbon emissions and environmental damage.
The carbon footprint of AI has been the subject of several studies, highlighting the significant environmental impact of this technology. At ACL 2019, the research "Energy and Policy Considerations for Deep Learning in NLP" was presented. It states that training a single deep-learning model can emit up to 284,000 kg of CO2; this is equivalent to the energy consumption of five cars over their lifetime. Other studies have similarly highlighted the environmental impact of AI, particularly in terms of its energy consumption and carbon emissions.
The scale of the negative environmental impact of AI is significant, with one study suggesting that the carbon emissions associated with AI are equivalent to the entire airline industry's emissions. It is projected that the energy consumption of AI will increase by up to 300% by 2025, with data centres and their associated infrastructure accounting for most of this energy consumption. This highlights the urgent need to address the environmental impact of this technology and find more sustainable ways to develop and deploy AI.
ChatGPT: Energy and Cost Consumption of Large Language Models
Large language models like ChatGPT are among AI's most computationally expensive applications. Training these models requires significant computing resources, including specialised hardware such as graphics processing units (GPUs) and tensor processing units (TPUs). The energy consumption associated with training large language models is significant and has been a topic of concern for environmental sustainability.
A recent study by researchers at the University of Massachusetts Amherst estimated the energy consumption associated with training several large language models, including OpenAI's GPT-2 and GPT-3. The study found that training these models required energy consumption in the range of hundreds of thousands to millions of kWh, with corresponding greenhouse gas emissions in the range of tens of thousands to hundreds of thousands of kg of CO2.
The energy consumption associated with training large language models is significant, and it seems that a single prompt in ChatGPT consumes 100x more energy than a single Google search. According to Sam Altman, a single prompt costs “probably single-digits cents” in energy, which could mean a 0,3 kWh per request, versus a 0.0003 kWh per Google search.
This will require a concerted effort from governments, businesses, and individuals to minimise the negative environmental impact of AI while harnessing its potential to enhance sustainability efforts.
AI and e-Waste
AI's production and disposal of e-waste generated by its supporting infrastructure is a growing environmental concern. A crucial component of a long-term approach to sustainable AI is the need to address the issue of e-waste. AI technologies rely on complex hardware components that can be difficult and expensive to recycle or dispose of safely. As the demand for AI systems continues to grow, so too does the amount of e-waste generated, with potentially significant environmental and health impacts.
E-waste can contain toxic chemicals and materials, leading to pollution and other environmental damage. This further exacerbates the environmental impact of AI and highlights the need for more sustainable approaches to the design and deployment of AI infrastructure. To address this challenge, we need to design AI systems that are more sustainable from the outset, with a focus on recyclability, durability, and repairability.
Fortunately, efforts are already underway to reduce the carbon footprint of AI. Researchers and industry leaders are exploring ways to reduce the energy consumption and carbon emissions associated with AI. This includes using more energy-efficient hardware and software solutions, such as low-power processors and more efficient algorithms. It also involves the development of more sustainable data centre infrastructure, including using renewable energy sources to power these facilities.
The Potential of AI for Sustainability
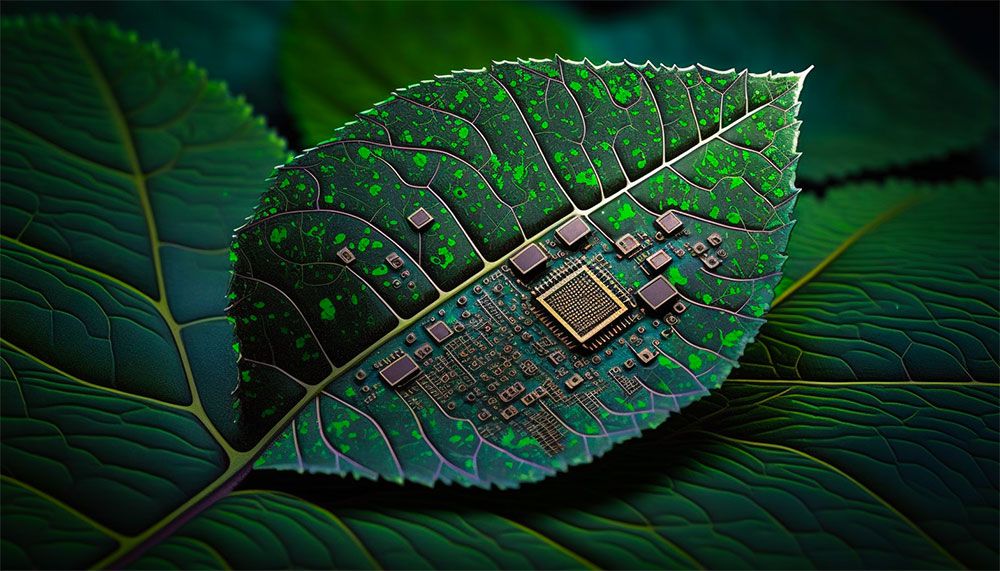
AI has the potential to revolutionise the way we address sustainability challenges. With its ability to analyse vast amounts of data and identify patterns, AI can help us make more informed decisions about resource management, energy efficiency, and pollution reduction. By automating repetitive tasks, AI can also free up human capacity to focus on more creative and strategic approaches to sustainability. However, AI is not a silver bullet solution. Its deployment must be guided by ethical considerations to ensure it benefits all stakeholders and does not exacerbate existing social and environmental inequalities.
From improving energy efficiency to enhancing resource management, AI is being deployed in a wide range of applications to support sustainable development. In this section, we will explore some case studies of sustainable AI, highlighting innovative uses of AI to address environmental challenges and promote sustainability. These examples demonstrate how AI can be harnessed to create a positive environmental impact and generate economic and social benefits.
Monitoring Deforestation
AI is being used to monitor deforestation activities in various parts of the world. For instance, in Brazil, the Rainforest Connection has developed a system that uses AI and acoustic sensors to detect and report chainsaw noises, commonly used in illegal logging. This technology could help reduce deforestation in the Amazon rainforest by up to 71% in some areas.
Similarly, Global Forest Watch uses AI and machine learning algorithms to analyse satellite imagery and track changes in forest cover. This platform helps identify areas at risk of deforestation and enables policymakers and conservationists to take timely action.
Detecting Illegal Fishing and Water Management
Illegal fishing is a major threat to marine ecosystems, and AI is being used to detect and prevent such activities. For example, the OceanMind project uses satellite imagery, vessel tracking data, and AI algorithms to monitor fishing activities and identify illegal, unreported, and unregulated fishing vessels. This project has helped reduce illegal fishing in West Africa and Southeast Asia waters.
In addition, the Global Fishing Watch project uses AI and machine learning algorithms to analyse vessel movements and track fishing activities in near real-time. This platform enables authorities to detect and prevent illegal fishing activities, thus protecting marine ecosystems and ensuring the sustainability of fish stocks.
AI can also be used in the water industry to better monitor and manage water resources. By analysing data from sensors and other sources, AI can help identify leaks, predict water usage patterns, and optimise water management systems, reducing water waste and conserving this vital resource.
Sustainable Agriculture
AI is being used to optimise agricultural practices and reduce waste. For example, the startup Taranis uses AI and computer vision to analyse crop images and identify potential issues such as nutrient deficiencies, pests, and diseases. This technology helps farmers to take targeted actions to improve crop health and yield while reducing the need for pesticides and other inputs.
AI can also help in managing and optimising waste management systems. It can be used to monitor landfills and identify areas where recycling and composting programs can be implemented, reducing the amount of waste sent to landfills and minimising the environmental impact.
Commercial buildings and construction
AI is also used to optimise renewable energy systems and improve energy efficiency. For instance, the startup Verdigris uses AI algorithms to analyse energy consumption patterns in commercial buildings and identify areas where energy waste can be reduced. This technology has helped businesses to reduce their energy bills by up to 20%. AI can also help to optimise energy use in buildings, reducing energy consumption and greenhouse gas emissions.
In the construction industry, AI can help reduce the environmental impact of building materials. By analysing data on the lifecycle of materials, AI can help identify more sustainable alternatives, reducing the environmental impact of construction.
Climate Modeling
This is another industry in which AI is being used to improve climate models and make more accurate predictions about the impacts of climate change. The Climate Informatics project uses machine learning algorithms to analyse climate data and identify patterns that can be used to improve climate models. This technology can potentially improve our understanding of climate change and inform policy decisions to mitigate its impacts.
Green Energy Initiatives for AI Infrastructure
Several renewable energy solutions, energy-efficient hardware designs, and other cutting-edge initiatives have the potential to revolutionise the AI industry while reducing its carbon footprint.
Let's look at some of the most relevant approaches to tackle the issues related to AI's carbon footprint. One example is using renewable energy to power data centres, which are essential to AI. Several companies, including Google and Microsoft, have committed to using 100% renewable energy to power their data centres, reducing the carbon footprint of these facilities.
Another approach is to use more energy-efficient hardware for AI. This includes designing AI-specific chips that consume less power and optimising software to reduce energy consumption. This approach can significantly reduce the energy required to run AI applications, thus reducing the environmental impact.
Edge computing is also being explored as a way to reduce the energy consumption of AI. Edge computing involves performing AI computations on devices at the network edge, such as smartphones and IoT devices, rather than on centralised servers. This method reduces the need for data to be transferred to and from centralised servers, reducing energy consumption and latency.
Efforts are also being made to reduce the environmental impact of training deep learning models, which can be resource-intensive and emit significant CO2. One approach is to use more efficient algorithms that require less computational power and time to train models. Researchers are actively working to develop more efficient algorithms that require fewer computational resources and, therefore, less energy. One promising method is the development of "sparse" neural networks, which reduce the number of connections between neurons and minimise the computational load required to train the network. Another promising avenue of research is transfer learning, which involves using pre-trained models to improve the accuracy and efficiency of new models, reducing the overall energy consumption required to train new models from scratch.
With that said, efforts to use more green energy in AI infrastructure are ongoing, with companies and researchers exploring various approaches to reduce the environmental impact of this technology. These efforts are crucial to ensuring that AI can be used sustainably and responsibly, contributing to a more sustainable future.
Download the 2023 Technology Trends eBook
Written by Strategic Futurist and Innovation Speaker Dr Mark van Rijmenam
The Future of Sustainable AI
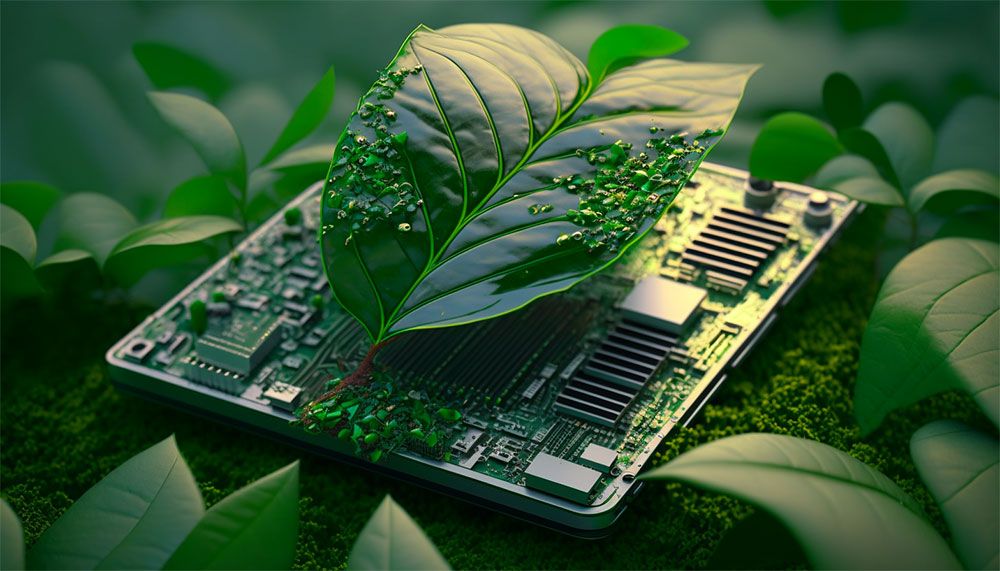
As we continue to grapple with the complexities of achieving sustainability in the face of global environmental challenges, the potential of artificial intelligence to support sustainable development is becoming increasingly clear. This enables us to make more informed decisions about resource management, energy use, and pollution reduction. However, to fully realise the potential of sustainable AI, we must also consider the ethical implications of this technology.
The issue of data privacy is directly relevant to AI efforts to protect the environment, as it affects the collection and use of environmental data. Many AI applications that aim to protect the environment, such as monitoring deforestation or tracking animal populations, rely on large amounts of data to function effectively. This data often includes sensitive information about individuals, communities, and ecosystems, and it is crucial to ensure that it is collected and used ethically and securely. In low-income countries, where there may be a lack of strong data protection laws and regulations, there is a risk that environmental data could be collected, stored, or processed in ways that are not ethical or secure. This could undermine public trust in AI's ability to protect the environment and hinder its development in low-income countries.
Therefore, it is essential to address the issue of data privacy as part of sustainable AI development efforts aimed at protecting the environment. By ensuring that environmental data is collected ethically and with the consent of those providing the data and that strong data protection laws and regulations are put in place and enforced, we can build a sustainable and trustworthy AI industry that supports environmental protection efforts. We can harness the power of AI to address pressing environmental challenges while respecting individuals' rights to privacy and security.
Another important consideration is the need for ongoing monitoring and evaluation of sustainable AI solutions. We must continuously assess their impact on the environment, society, and the economy to ensure AI achieves its intended goals and does not inadvertently contribute to new challenges. This requires developing robust monitoring and evaluation frameworks that consider a wide range of indicators and metrics.
A Long-term Approach to Sustainable AI
Sustainable AI is more than just a buzzword; it represents a long-term approach to developing artificial intelligence systems that can meet the needs of the present without compromising the ability of future generations to meet their own needs. As we continue to rely on AI to solve complex problems and drive innovation, it is critical to adopt a long-term perspective that takes into account the social, economic, and environmental impact of our actions.
A long-term approach to sustainable AI requires a fundamental shift in our approach to innovation, one that values collaboration and community-driven problem-solving over narrow technological advances. To create AI systems that are truly sustainable, we need to bring together experts from diverse fields, including environmental science, social science, engineering, and computer science, to co-create solutions that are technically advanced, socially responsible, and environmentally sustainable for our future generations. This collaboration can help ensure that sustainable AI solutions are developed with a deep understanding of the complex social and environmental systems they are intended to address. For example, engineers and computer scientists are developing specialised AI chips optimised for low power consumption and exploring ways to optimise the software algorithms used to train and run AI models.
Finally, we must consider the potential unintended consequences of sustainable AI solutions. As with any technology, there is a risk that AI could exacerbate existing environmental or social challenges if not developed and deployed responsibly. Increased resource consumption—since AI solutions may require additional resources to develop and maintain, such as renewable energy sources, specialised hardware, or new data collection processes— trade-offs between sustainability and efficiency, unintended consequences of algorithmic decision-making, and job displacement and inequality are some of the potential challenges to consider. It is important to anticipate and mitigate these risks by carefully considering potential scenarios and proactive measures to address any negative impacts.
All in all, the future of sustainable AI holds tremendous promise but also presents significant challenges. By taking a comprehensive approach to sustainable AI development and deployment, we can maximise the potential of this technology to support sustainable development and create a more equitable and sustainable future for all.
Final Thoughts
Sustainable AI has the potential to play a crucial role in addressing the pressing sustainability challenges we face as a society. From mitigating climate change to promoting sustainable transportation, agriculture, and circular economy practices, AI can help us create a more sustainable future for our planet.
However, it is important to approach the development and deployment of AI technologies with a responsible and ethical mindset. As AI becomes increasingly ubiquitous in our daily lives, it is essential to ensure that these technologies are developed in a way that prioritises environmental sustainability, social responsibility, and ethical considerations. This includes minimising energy consumption, reducing greenhouse gas emissions, and promoting transparency and accountability in AI development and deployment.
As AI evolves and becomes more sophisticated, we must engage in open and transparent conversations about the opportunities and challenges associated with sustainable AI. By working together to create a more sustainable and responsible future for AI, we can harness the full potential of this transformative technology to create a more equitable, just, and sustainable world for all.
Images: Midjourney