Garbage In is Garbage Out; How Big Data Scientists Can Benefit from Human Judgment
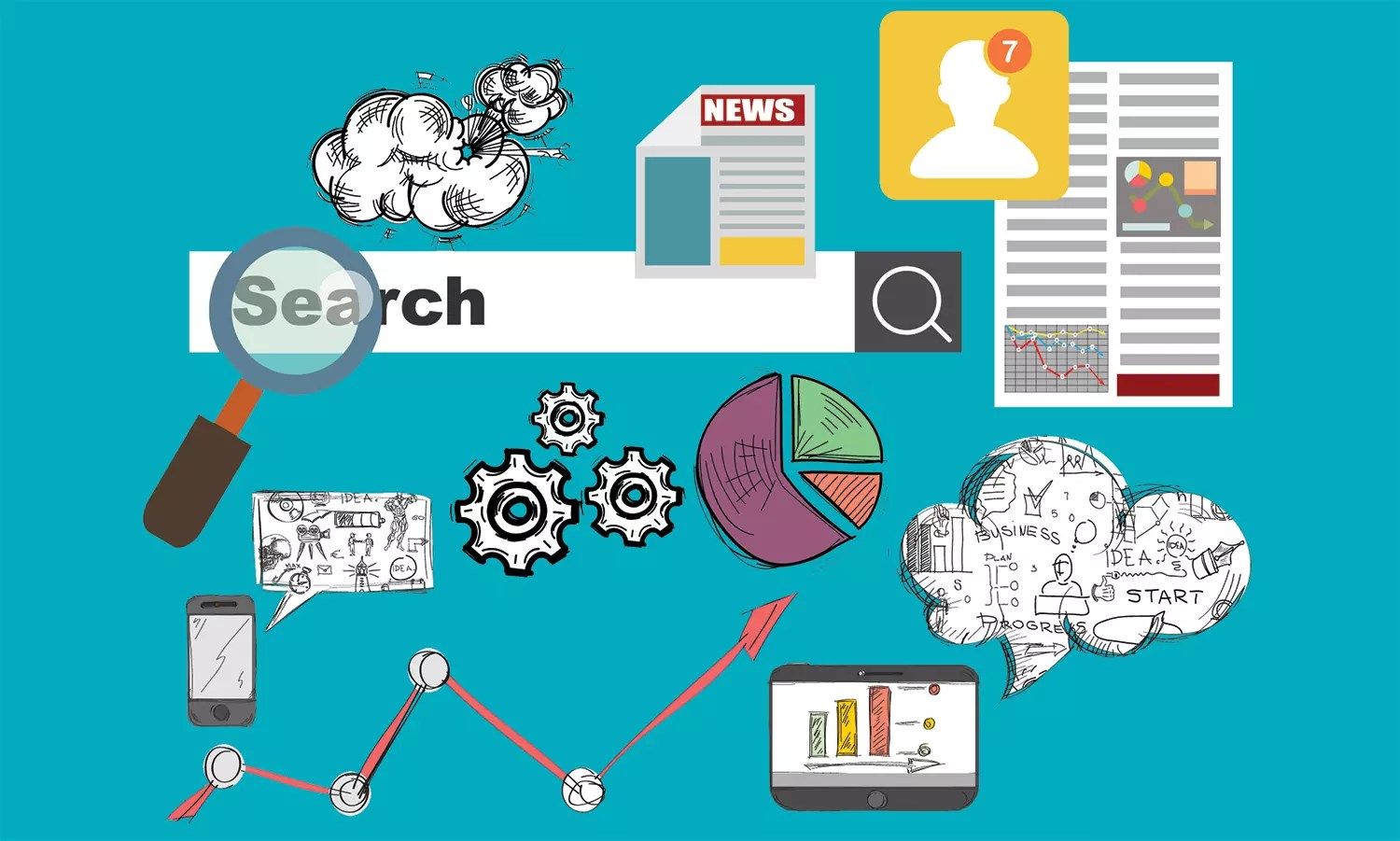
The quality of your data determines the quality of your insights from that data. Of course, the quality of your data models and algorithms have an impact on your results as well, but in general, it is garbage in, garbage out. Therefore, (Total) Data Quality Management (DQM) or Master Data Management (MDM) have been around for a very long time and it should be a vital aspect of your data governance policies.
Data governance can offer many benefits for organizations, including reduced headcount, higher quality of data, better data analysis and time savings. As such, those companies that can maintain a balance of value creation and risk exposure in relation to data can create competitive advantage.
Human judgments and Data Quality
Garbage in, garbage out. Especially with the hype around artificial intelligence and machine learning, that has become more important than ever. Any organization that takes itself serious and employs data scientists to develop artificial intelligence and machine learning solutions should take the quality of data very serious. Data that is used to develop, test and train algorithms should be of high quality, high volume and should be well organised and enriched. The right high-quality data will result in better and smarter algorithms that will return better insights and results.
The problem is, however, that improving and optimising your data to develop better machine learning algorithms is difficult, time-consuming and very expensive if done by highly-talented data scientists. While it is a necessity, it does not have to cost the world and if done correctly, can actually free-up your data scientists to focus on developing better machine learning algorithms. How, you might wonder? By turning to the crowd to help you train your algorithms with the right high-quality data, that is cleaned, parsed and enriched.
The Importance of Human judgments
A few years ago, Andrew McAfee proposed a simple rule for the second machine age: as the amount of data goes up, the importance of human judgment goes down. McAfee argues that we should turn most of our decisions over to algorithms; algorithms that have access to vast troves of data and are based on mathematical models which are not bothered by human traits such as emotions or feelings. As such, algorithms are a lot better at making informed decisions than humans. But, McAfee also argues that when expert opinions are quantified and added to the algorithm, the quality of the outcome generally goes up. As such, human judgment becomes important as an input to algorithms, but not as a replacement for algorithms.
Practically speaking, human judgments, as part of the input for algorithms, have increasingly become important for data scientists to develop good algorithms and models. Human judgment is, in fact, essential at the initial design stages of the models. Algorithms require human interpretive skills to improve the outputs of the machine learning models and human judgment can help improve the quality of the data and as such improve the outcome of the model. Although McAfee argued that the importance of human judgments goes down, in fact, it has become more important than ever. Next to human judgments becoming more important, the data governance is also increasingly seen as an area for competitive advantage.
The Importance of Data Governance
Data governance enables firms to comply with regulations and proper data governance policies can offer you a competitive advantage. Data governance comprises several important areas: data principles, data quality, metadata, data access and data lifecycle:
Data principles define how business users can manage and deal with the data available. Data quality refers to the accuracy, timeliness, credibility and completeness of the data. Metadata is defined as “data about data” and provides a description of data to facilitate the understanding of data. Data access determines who has access to what data within the organization and data life cycle is about how data is used, stored and organised over time. In addition, data governance is viewed as those policies, processes and organizational structures that enable data valuation; accessibility, monitoring and recovery of data; as well as ownership and stewardship.
However, many organizations lack a clear understanding of the value of their data or don’t see data as an asset. As a result, companies could face a variety of problems that could harm the business, including inconsistencies in data definitions, formats and values that make it difficult for organizations to understand and use their data, which could result in significant problems, and as such loss in time and money, when building data models.
Decades of using and storing data in disparate stores and formats have resulted in many irregularities making it difficult for companies to understand their data. Hence, organizations that do focus on high-quality data are better able to deal with changing business environments and achieve strategic objectives. As such, organizations need data quality management processes that enable high-quality corporate data, resulting in a “single version of the truth” to cope with the strategic and operational challenges of their environment. In today’s data-driven world, data governance has, therefore, become a necessity for organizations and they cannot get away anymore with minimal effort. It has to become an integral part of their processes and good big data scientists take data quality very seriously and often have to spend a lot of time fixing the data.
However, cleansing, parsing or enriching data and improving the quality of the data is not what data scientists should be doing. They should be focusing on building models and writing/testing/improving algorithms, the fun things, the tasks that data scientists enjoy and are good at. They should not spend time on tasks that take up too much of their, often expensive, time. However, the work is very important for organizations (remember: garbage in is garbage out) and luckily there are other ways to go about.
Full Stack Human Judgments
Several organizations offer access to the crowd to help data teams collect, clean, enrich and label data at scale to make it useful for your data scientists. However, there is a lot more to it than simply turning to a crowdsourcing vendor to do this. It involves informing the crowd of your intentions, managing the crowd, monitoring the crowd and eventually evaluating the crowd and the work was done to ensure that you obtain precise and clean training and evaluation data that data scientists can use with high-confidence.
That’s where full stack human judgments come in, to assist internal teams of data scientists and offer them the right data for the right data scientists. One of such organizations that offers a Data Science Support program is Search Strategy Solutions. They have developed a program to offer your data scientists with high-quality, reliable human judgments and data to support them in developing great machine learning algorithms.
In the highly-competitive world that we live in, nowadays, it becomes more and more important to use artificial intelligence and machine learning to create better products and services and develop solutions that benefit your customers. The right machine learning algorithms can help you remain competitive, but only if your algorithms are trained with high-quality data and work as they should be. That’s why any organization that works with data and machine learning algorithms, should pay attention to cleaned, enriched and high-quality data. organizations such as Search Strategy Solutions can help you achieve that.
Image credit: Tetiana Yurchenko/Shutterstock