Five Data Mining Techniques That Help Create Business Value
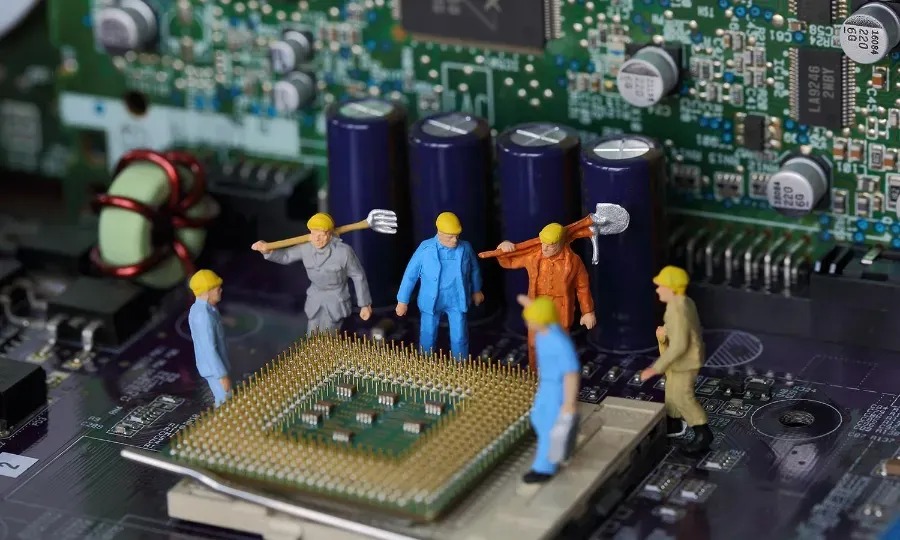
There are many different types of analysis to retrieve information from big data. Each type of analysis will have a different impact or result. The data mining technique you should use, depends on the kind of business problem that you are trying to solve.
Different analyses will deliver different outcomes and thus provide different insights. One of the common ways to recover valuable insights is via the process of data mining. Data mining is a buzzword that often is used to describe the entire range of big data analytics, including collection, extraction, analysis and statistics. This, however, is too broad as data mining refers to the discovery of previously unknown patterns, unusual records or dependencies. When developing your big data strategy, it is important to have a clear understanding of what data mining is and how it can help you.
The term data mining first appeared in the 1990s while before that, statisticians used the terms “Data Fishing” or “Data Dredging” to refer to analysing data without an a priori hypothesis. The most important objective of any data mining process is to find useful information that is easily understood in large data sets. There are a few important classes of tasks that are involved with data mining:
Anomaly or Outlier Detection
Anomaly detection refers to the search for data items in a dataset that do not match a projected pattern or expected behaviour. Anomalies are also called outliers, exceptions, surprises or contaminants and they often provide critical and actionable information. An outlier is an object that deviates significantly from the general average within a dataset or a combination of data. It is numerically distant from the rest of the data and, therefore, the outlier indicates that something is out of the ordinary and requires additional analysis.
Anomaly detection is used to detect fraud or risks within critical systems and anomalies have all the characteristics to be of interest to an analyst, who should further analyse it to find out what’s really going on. It can help to find extraordinary occurrences that could indicate fraudulent actions, flawed procedures or areas where a certain theory is invalid. Important to note is that in large datasets, a few outliers are common. Outliers may indicate bad data but may also be due to random variation or may indicate something scientifically interesting. In all cases, additional research is required.
Association Rule Learning
Association rule learning enables the discovery of interesting relations (interdependencies) between different variables in large databases. Association rule learning uncovers hidden patterns in the data that can be used to identify variables within the data and the co-occurrences of different variables that appear with the greatest frequencies.
Association rule learning is often used in the retail industry when to find patterns in point-of-sales data. These patterns can be used when recommending new products to others based on what others have bought before or based on which products are bought together. If this is done correctly, it can help organisations increase their conversion rate. A well-known example is that of Walmart’s Strawberry Pop-tarts. Thanks to data mining, Walmart, already in 2004, discovered that Strawberry Pop-tarts sales increased by seven times before a hurricane. Since this discovery, Walmart places the Strawberry Pop-Tarts at the checkouts before a hurricane is about to happen.
Clustering Analysis
Clustering analysis is the process of identifying data sets that are similar to each other, to understand the differences as well as similarities within the data. Clusters have certain traits in common that can be used to improve targeting algorithms. For example, clusters of customers with similar buying behaviour can be targeted with similar products and services to increase the conversation rate.
A result from a clustering analysis can be the creation of personas. Personas are fictional characters created to represent the different user types within a targeted demographic, attitude and/or behaviour set that might use a site, brand or product in a similar way. The programming language R has a large variety of functions to perform relevant cluster analysis and is therefore especially relevant for performing a clustering analysis.
Classification Analysis
Classification Analysis is a systematic process for obtaining important and relevant information about data, and metadata - data about data. The classification analysis helps to identify the categories the data belongs. Classification analysis is closely linked to cluster analysis as the classification can be used to cluster data.
Your email provider performs a well-known example of classification analysis: they use algorithms that can classify your email as legitimate or mark it as spam. This is done based on data that is linked with the email or the information that is in the email, for example, certain words or attachments that indicate spam.
Regression Analysis
Regression analysis tries to define the dependency between variables. It assumes a one-way causal effect from one variable to the response of another variable. Independent variables can be affected by each other, but it does not mean that this dependency is both ways as is the case with correlation analysis. A regression analysis can show that one variable is dependent on another but not vice-versa.
Regression analysis is used to determine different levels of customer satisfactions and how they affect customer loyalty and how service levels can be affected by for example the weather. A more concrete example is that a regression analysis can help you find the love of your life on an online dating website.
Data mining can help organisations and scientists to find and select the most important and relevant information. This information can be used to create models that enable predictions how people or systems will behave so you can anticipate on it. The more data you have, the better the models will become that you can create using the data mining techniques, resulting in more business value for your organisation.