How Data Lakes Open New Possibilities for Your Organisation
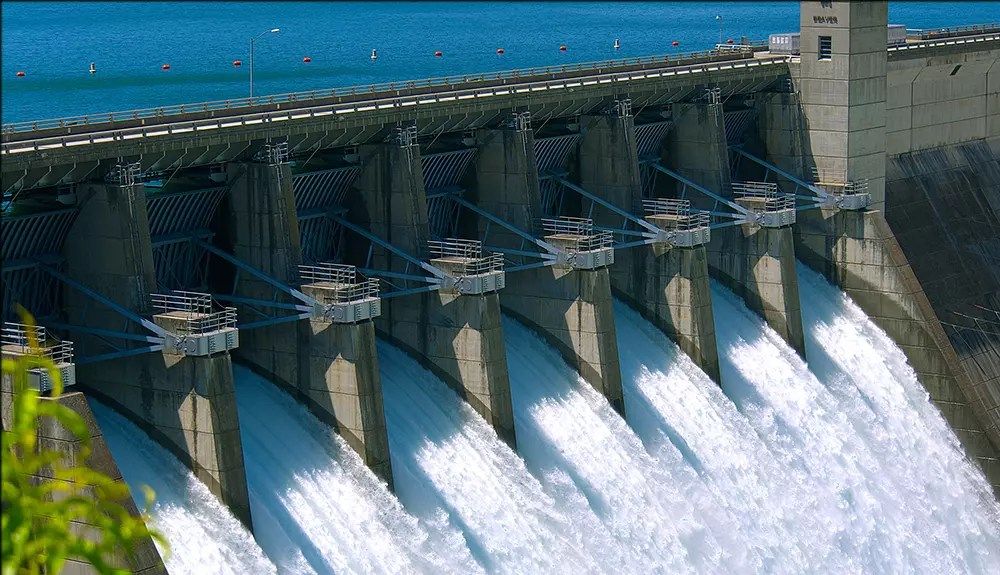
In the past years, I have seen a flood of information on data lakes, and it looks like it is becoming as much of a buzzword as Big Data. I described data lakes as a growing trend in 2015 – stating that it was time for organisations to experiment with data lakes. But are we seeing much progress?
Sure, organisations like Facebook, Google and Yahoo have advanced considerably, and their developers experience numerous benefits using data lakes. But what does it mean – a data lake – and what are the benefits of it? And are ‘offline’ organisations such as retailers and financial services companies also moving towards a data lake model? What are the advantages and challenges of a data lake and how can you derive value from it? I think it’s time for a deep dive into data lakes.
The Data Lake Definition
But first, what exactly is a data lake. Data lakes are defined as a repository containing vast amounts of raw data, in native formats, while allowing different users to access and analyse that data as required. A data lake enables an organisation to easily connect enterprise data, capturing, mixing and exploring new types of data to derive new, and more, value from their data.
A data lake enables organisations to bring together all kinds of data, combine the data sources, generate meaning from it and, of course, derive value out of it. Earlier I wrote about the concept of Mixed Data, which entails the concept of combining different data sources to derive insights. Mixed Data benefits significantly from data lake architectures.
According to PWC, with a data lake, developers and programmers can easily tap into the streams of data present in the data lake for analysis, while data scientists can use a data lake for discovery and ideation. In addition, a data lake can serve as a staging environment for more sensitive, or ‘treated’ data that will be stored in a data warehouse.
Five Advantages of a Data Lake
Every organisation or industry can benefit from a data lake and create the use case for it. A data lake can be used to end the data silos within your organisation, centralise the data and gain better access to all disparate data sources within your business.
Popular use cases include achieving 360-degree views of customers, and analysing social media, but it also enables healthcare organisations to optimise treatments and enables manufacturers to derive insights from sensor data. The advantages of data lakes are numerous:
1. Low Cost, Extremely Scalable Storage
The costs for storing data in a data lake are low, and it can easily scale to extreme volumes.
2. Supporting Multiple Programming Languages and Frameworks
Thanks to the raw data that is stored in the data lake, developers can work with multiple programming languages such as Python or Java and use different frameworks such as Hive or Pig.
3. Data Agnostic and Immediate Access to All Data
A data lake can contain any data, from structured machine data to unstructured social media data in one central location. In addition, users have immediate access to all data, since it is in one location, which of course should be role-based.
4. Centralised Data That Does not have to Be Moved
With a data lake, all your data is in one central location. Silos are no longer necessary, making it easier to access and mix the different data sources. In addition, it is no longer necessary to move the data from one warehouse to another.
5. More Insights Due to Raw Data
With a data lake, organisations can store the data in raw format, meaning that no information is lost along the way. In the future, as additional opportunities to leverage the data arise, companies can go back to the original data for answers.
Four Challenges of Data Lakes
Of course, data lakes are not all only positive. There are some serious challenges that need to be solved before data lakes will be adopted on a large scale:
Meta Data Management
A data lake is only truly valuable to an organisation if its data is tagged and catalogued. Tagged data ensure better queries and better analysis. For example, metadata is a vital component of a data lake. Metadata provides context, which is vital in a data-driven world where we have data from multiple sources.
Twitter is very good at this. In fact, each tweet collects 65 data elements used to provide context for each tweet. Metadata enables us to combine and mix data to achieve insights that will transform your business. Unfortunately, applying the right metadata at the right moment to the right data within the data lake can be a challenge. Adding metadata as soon as the data is entered in the data lake is a best practice, but it is not a common practice. Furthermore, there are not many tools available on the market that can assist in that.
One of the companies that actually has developed a system of automatically adding the right metadata upon data ingestion in the lake is Zaloni. Their product, which is called Bedrock, provides significant automated metadata capabilities that can enrich your raw data and provide the context required to make the most of your data, thereby solving a big challenge linked to current data lakes.
Data Governance
Data governance is a challenge for any organisation dealing with data in general and big data specifically. But when it comes to data lakes, this becomes even more important. If data governance is not taken care off when starting with a data lake, you can enter ‘data limbo land’ where all kinds of issues related to data quality, metadata management or security could arise, causing your data lake to fail dramatically.
The right processes should be in place within your organisation to ensure that data governance is done correctly and to ensure that the right data is stored correctly and the right and correct algorithms are used to analyse your data.
Data Preparation
Another challenge is to ensure proper dealing with the data. As more democratised access to the lake is allowed, and self-service becomes more common, finding ways to address data quality and preparation becomes ever more critical.
There are two distinct options to ensure proper data preparation: 1) employ the right data scientists who understand how to create sophisticated analytics models while ensuring data quality and data lineage or 2) use a system that prepares the raw data (semi-) automatically and thereby enable end-users to easily query the data and/or import in different analytical tools to gain insights from the data.
The first option might not be suitable, because hiring, or training, data scientists is expensive and sometimes that is not possible for an organisation. The second option becomes more and more available since Big Data vendors are developing tools to do this automatically.
One of these tools is Mica, which enables self-service data preparation enabling end-users to work with the raw data how they want, collaborate with the data and analyse it using different analytical tools. The main advantage is that business users don’t have to wait until IT has done the data preparation, because they can do it themselves. Mica even enables users to operationalise the data preparation so that it will happen automatically when relevant new data enters the lake.
Data Security
Of course, when you have all data in one central location, security becomes an issue. Already too many organisations have experienced data breaches, and when your data lake is breached, it seriously could mean the end of your business. Therefore, the ‘standard’ security measures should be in place.
However, having your data stored in one place has an additional security issue. Therefore, role-based access is of extreme importance when building a data lake. The data lake should ensure that although the data is stored in one central location, access is determined based on the role you have. To enforce this, you can for example tag metadata with security data to ensure that role-based access is also implemented on the metadata level.
Deriving Significant Value from Your Data Lake
Of course, the objective of building a data lake is to derive value from it. If done correctly, having your data stored in a single repository and being able to easily analyse the raw data with existing data analytics tools, will provide your organisation with significant new insights.
Mixing different data sources and analysing them opens up new possibilities and with a data lake, that process all of a sudden becomes a lot easier. Therefore, organisations that have implemented a data lake will reap the benefits from it in the future.
Image: FeyginFoto/Shutterstock